Unlocking the Future of Business with Data Annotation in Machine Learning
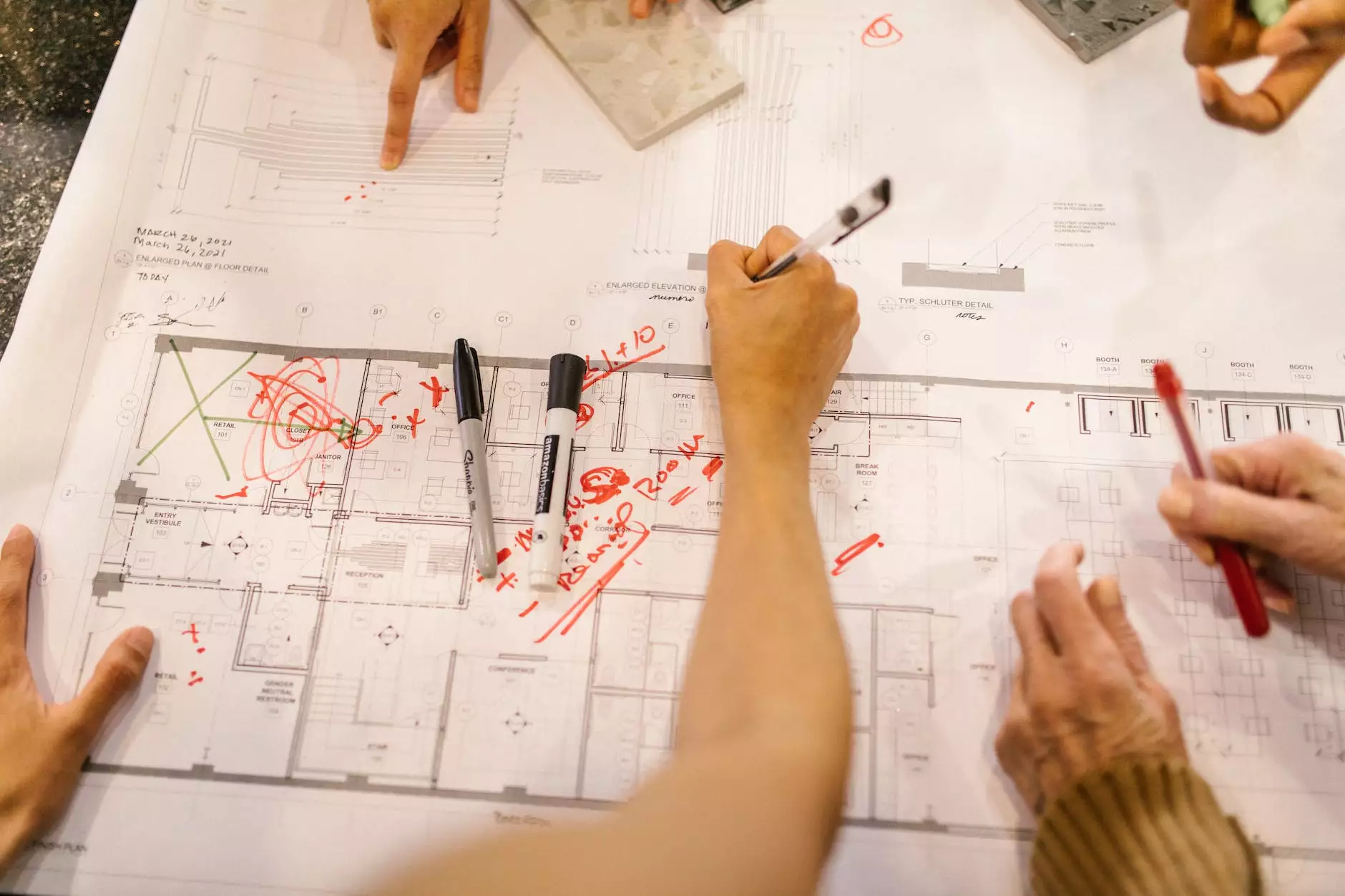
In today’s rapidly evolving digital landscape, data annotation in machine learning stands out as a pivotal component for businesses aiming to leverage artificial intelligence to gain a competitive edge. This article delves into how proper data annotation enhances various industries, including home services, keys, and locksmiths. With understanding its importance, businesses can unlock new efficiencies, accuracy, and customer satisfaction.
Understanding Data Annotation in Machine Learning
At its core, data annotation is the process of labeling data for machine learning algorithms to learn from. This can involve categorizing images, transcribing audio, tagging parts of text, or any other tasks that prepare data for training AI models. Machine learning thrives on high-quality, well-annotated datasets as it enables the model to improve its predictions and validate its outputs.
The Importance of Data Annotation
1. Enhanced Accuracy and Efficiency
High-quality data annotation is crucial for businesses aiming for precision. In sectors such as home services, an accurately annotated dataset allows companies to better understand customer needs, predict demand, and manage resources efficiently. For locksmiths, data regarding typical customer inquiries and service requests can streamline operations, ensuring timely services.
2. Building Robust AI Models
Businesses leveraging machine learning must feed their algorithms with properly annotated data. The accuracy of AI predictions heavily depends on the quality and consistency of the data annotations. A well-trained model can identify trends, automate processes, and ultimately improve decision-making.
3. Boosting Customer Satisfaction
When machines understand customer behavior and preferences through well-annotated data, they can provide more personalized experiences. Whether it’s timely responses from a locksmith or customized home service offers, customer satisfaction is directly influenced by how well businesses utilize data annotation in machine learning.
Key Techniques for Data Annotation
1. Manual Annotation
This is the traditional method wherein human annotators meticulously label data. Manual annotation is often needed for complex tasks where context is essential, such as understanding customer emotions in text or intricate scenarios in images. For a locksmith business, this could mean accurately identifying the specifics of different types of locks in training datasets.
2. Automated Annotation
With advancements in technology, some data annotation tasks can now be automated using algorithms and AI tools. While not as precise as manual annotation, this method significantly speeds up the labeling process and is particularly useful for smaller, less complex datasets.
3. Crowd-sourced Annotation
This technique involves outsourcing data labeling tasks to a large group of individuals, often through online platforms. While cost-effective and fast, crowd-sourced annotation requires strong quality control measures to ensure that the data labels are accurate and reliable.
Benefits of Using Data Annotation in Home Services and Locksmiths
The integration of data annotation into businesses, specifically in home services and locksmithing, brings notable advantages:
- Improved Marketing Strategies: By analyzing annotated data related to customer interactions, businesses can design targeted marketing campaigns that resonate with potential customers.
- Streamlined Operations: Data annotation can help identify operational inefficiencies, recommending areas for improvement to deliver services more efficiently.
- Predictive Analysis and Forecasting: Annotated datasets enable advanced analytics that can predict future trends, giving businesses an upper hand in their strategic planning.
- Enhanced Customer Insights: Businesses can analyze detailed data to gain insights into customer needs, which can drive product development and service improvements.
Challenges in Data Annotation
1. Quality Control
One of the biggest challenges in data annotation is maintaining high-quality standards. Inaccurate labels can lead to poorly trained models, resulting in ineffective AI systems. Businesses must establish stringent protocols for quality control to ensure data integrity.
2. Time-Consuming Processes
Although automation is a promising avenue, manual annotation remains a time-consuming endeavor. Balancing the need for speed with the necessity of quality can be a significant bottleneck for businesses.
3. Skilled Resources
Finding skilled annotators who understand the context of the data is crucial. For specialized fields, like locksmithing, it's vital that annotators comprehend the nuances of the industry to label data accurately.
Future Trends in Data Annotation for Businesses
As businesses look to the future, several trends are emerging in the realm of data annotation that could significantly impact home services and locksmith industries:
- Increased Use of AI in Annotation: AI-driven annotation tools are expected to improve in accuracy and efficiency, reducing the workload on human annotators and increasing overall productivity.
- Focus on Privacy and Ethics: As more data is collected, businesses will need to prioritize ethical data annotation practices and ensure compliance with privacy laws.
- Customized Annotation Solutions: Companies will likely seek tailored annotation solutions that cater specifically to their unique industry needs rather than one-size-fits-all approaches.
Conclusion
In conclusion, the power of data annotation in machine learning cannot be overstated, especially for businesses operating in the home services and locksmith sectors. By leveraging well-annotated datasets, companies can enhance their operational efficiencies, better understand customer preferences, and ultimately deliver a superior service experience. As this technology continues to evolve, it will undoubtedly open new avenues for innovation and growth, making it imperative for businesses to incorporate robust data annotation processes into their strategies.
Investing in data annotation is not merely a tactical decision; it is a strategic necessity that will shape the future trajectory of any business striving for success in an increasingly data-driven world.
data annotation machine learning